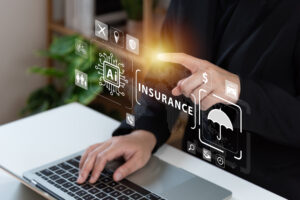
NAMIC examines what it calls insurance industry AI myths
By onAnnouncements
A new National Association of Mutual Insurance Companies (NAMIC) report warns that myths could be influencing regulations on the use of AI and emerging technology in the insurance industry.
“Particularly prevalent in insurance policy are myths about the effects of insurers using big data and artificial intelligence and unfounded notions about how insurance pricing should operate considering the argued effects,” the report says. “The danger in these myths is that there are growing numbers of advocates and regulators relying on them to advance policy that would ultimately harm the very population they are looking to protect: policyholders.”
AI creates systems and models that provide insights into data and are capable of providing or generating new content based on those insights and learning from large data sets, the report says. It adds that advanced data processing is beneficial to the insurance industry because it brings the prospect of increased risk insights and increased precision in risk-based pricing, which strengthens competition and benefits consumers.
Underwriting and ratemaking are used to create risk-based pricing, the report says. During underwriting the insurer asks:
-
- “How likely is it that the policyholder will experience a covered loss;
- “How should the risk be grouped with others like it (i.e., placing risks into groups based on common characteristics to discern expected loss probability); and
- “Do we want to take on this risk?”
The report outlines what NAMIC is describing as 5 “unfounded or false notions” surrounding insurance and AI, and also outlines how these concepts interact and
dispels misconceptions about them.
The first myth is that insurance can be regulated like other industries, the article says.
“Insurance products are uniquely priced prospectively based on risk and function differently than many other consumer products,” the report says. “Regulation specific to this foundation is therefore necessary.”
Insurance is often grouped with other industries, according to NAMIC, for regulations related to “algorithmic bias.” However, insurance does not have a standard price for his product, like most other industries, it says.
Insurers must make educated judgements about a policyholder’s expected risk of a covered loss. It says any regulation relative to AI and big data use in insurance risk-based pricing must be unique to the industry.
The second myth is that increasing risk-rating precision with AI and big data will create a ‘risk pool of one,’ which is detrimental to consumers.
“The more accurate risk rating is, the more insurers can take on riskier policyholders, thereby increasing availability,” the report says.
Competition among insurers drives the refinement of risk pools, the report says. A more accurate classification will more closely align premiums with the level of risk an insured presents.
Lower-risk individuals will be driven to insurers that have more refined risk classifications and lower corresponding premiums, the report said.
An argument against the increased precision has been that it could create an accessibility issue for high-risk insured.
“While competition for lower-risk insureds is generally more intense in the marketplace, insurers seeking to improve market penetration and economic advantage will necessarily compete for high-risk insureds,” the article says. “If an insurer can more accurately assess a risk, it can more accurately discern whether it can absorb a higher-risk insured. This fact, coupled with the economic drivers of market penetration, results in increased availability of insurance, even for high-risk individuals.”
NAMIC says a third myth is that fairness is one equal price for all consumers.
“Socialization of insurance will exacerbate issues of affordability that policymakers are concerned with today and not do anything to solve the underlying problems,” according to the report.
The report says that as a risk-priced product, any restriction on an insurer’s ability to price a policyholder’s risk will result in more, not fewer, access and affordability issues.
“Any discussions involving the industry’s use of AI, big data, and predictive analytics should embrace the risk-based history and industry foundation, both of which must be understood fully in the context of what constitutes fairness,” the report says. “To invoke or use a different standard of fairness, such as one that charges all groups similarly, would be catastrophic for policyholders, as it would increase premiums for all policyholders. It forces less-risky consumers to subsidize riskier consumers, which causes market distortions that affect affordability and availability of coverage for consumers, particularly the most vulnerable ones at the center of the fairness discussions being brought forth by some advocates.”
The fourth myth is that the use of AI and big data in risk-rating will lead to increased bias or separate impact and such outcomes should be prohibited.
“These concepts are incongruent with the risk-based foundation of insurance, where differential treatment of consumers is based on risk, not on protected class status,” the report says.
Insurance discriminates by risk types and charges premiums accordingly based on statistical differences, the NAMIC report says. Discrimination has a different, specific meaning in the business of insurance its regulatory state than in many other legal contexts.
“Separately, if one posits that bias means that the data is skewed or not representative, such concept of bias has not been proven or shown to be an issue in the context of algorithms, data use, or AI for insurance risk-based pricing. Bias, in this sense, results from incomplete or distorted data – for instance, a group that has been historically underrepresented in a particular context,” the report says. “In the context of insurance, however, this concept does not apply in the same way. As this paper has discussed, the data insurers use for risk-based pricing is data that is actuarially sound and correlated with risk and does not include nor use certain protected class attributes.”
The fifth risk is that Bayesian Improved First Name and Surname Geocoding (BIFSG) and multivariate analysis are useful tools in testing for algorithmic bias, the report says.
“Even if an outcomes-testing regime for bias were congruent with insurance and its legal frameworks, there are inherent limitations in reliable demographic data and testing methodologies for such purpose,” the report says.
Some states specifically prohibit insurers from collecting protected class data such as race or ethnicity, the report says. Any analysis of protected classes mandated by a regulator would require the industry to estimate an applicant’s or insured’s protected class status, a prospect that creates its own set of concerns and potential liabilities.
“It is important to note that BIFSG, while being inherently flawed, was not designed for use in an insurance regulatory context,” the report says. “It is also important to note that even if BIFSG were a reliable methodology for purposes of inferring race or ethnicity, there are not reliable methodologies for purposes of inferring other protected classes. To suggest that there will be other methodologies available in the future to infer protected class status of protected classes other than race is presumptuous at best and raises its own concerns of bias. For instance, it is difficult to contemplate how an insurer would predict someone’s religion or sexual orientation with any degree of confidence.”
The National Association of Insurance Commissioners (NAIC) approved a model bulletin on the use of AI by insurance companies in 2023.
The “Model Bulletin on the Use of Artificial Intelligence Systems by Insurers” outlines the need for processes and controls to prevent possible AI inaccuracies, discriminating biases, and data vulnerabilities.
The bulletin reminds insurers of established regulatory laws, such as the Unfair Trade Practices Model Act, that regulate unfair methods of competition or unfair or deceptive acts. It states governance and controls on AI systems are needed to comply with these laws.
“Compliance with these standards is required regardless of the tools and methods insurers use to make such decisions,” the bulletin says.
It also informs insurers about the steps state insurance departments could take to ensure that the companies follow the law. This could include documentation that insurers have set up governance and risk management frameworks for AI systems.
IMAGE
Photo courtesy of Poca Wander Stock/iStock