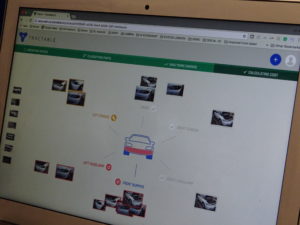
Tractable says data cleanup, volume, rules solve ‘GIGO’ problem of using past claims for AI photo estimating
By onBusiness Practices | Insurance | Market Trends | Repair Operations | Technology
Artificial intelligence photo estimating could check some of the worst behavior in the collision repair and auto insurance industries — or draw upon it to create an even worse scenario.
Given the level of sheer incompetence, refusal to follow or even look up OEM procedures, and elevation of speed or cost-cutting over a quality repair at times demonstrated by both industries, it seems likely that a large subset of claims handled by human insurers and repairers could underestimate, underrepresent or underpay the actual work done.
But teaching an AI to estimate claims by feeding in the photos and estimate data from those same vehicles and repairs would seem to raise the “Garbage In, Garbage Out” problem: A computer is only as good as the information fed into it. If that data is wrong, the computer will produce incorrect or distorted results.
That’s the question we put to Mitchell partner Tractable, which has taken hundreds of millions of photos paired with estimates and used them to create an artificial intelligence which can identify any external part of any vehicle, score its condition, and determine whether it ought to be repaired, replaced or left alone. The company estimates its system would be appropriate for up to 70 percent of claims — 50 percent involving external, nonstructural damage and another 20 percent classified as total losses.
Chief Commercial Officer Adrien Cohen agreed that basing Tractable’s photo-estimating computer purely on images and estimates alone would be problematic. But Tractable doesn’t stop there, he said. It has measures in place to ensure the computer picks what he described as the “safest and most efficient repair method.”
Estimate volume
The first is its sheer number of estimates, he said. A human adjuster only sees hundreds of claims a year, perhaps thousands, and thinks, “‘It doesn’t look that bad,'” Cohen said. But the AI, which has seen millions of claims, knows it is.
“We’re comparing it to the final estimate,” Cohen said. This includes cases where an unsupplemented initial estimate ended up being the final estimate and claims where a final estimate included supplements.
We asked why Tractable wouldn’t just throw out all of the claims without a supplement, given the potential for an initial estimate — particularly a photo estimate — to miss hidden internal damage. It’s possible the carrier or desk reviewer never performed a teardown, measured or scanned the vehicle, after all.
Cohen said including both one-and-done estimates and supplemented ones was the only way to ensure accuracy and create trust on both the insurer and repairer sides.
Beyond that, Tractable does a lot of supervision and training the AI on the information, rather than just feeding it into the machine and letting the machine be led astray by “noise” in the data, according to Cohen. The company also cleans the data “so it doesn’t learn the wrong things,” he said.
“That’s what we specialize in,” Cohen said. The company isn’t just “blindly pushing historical data,” he said.
Tractable also has a full-time body shop engineer on its staff. “I think that makes us very different,” he said.
But even with the in-house engineer, how can the computer rely on past data when vehicles are dramatically changing every design generation? What happens when Ford overnight switches the F-150 to aluminum, rendering years of steel F-150 estimates irrelevant? And how do you compensate when shops and insurers realize, as happened in 2016, that they’ve for more than a decade of estimates been failing to perform the necessary scans?
Cohen pointed out that Tractable isn’t relying solely on historical data.
“We receive new claims every day,” Cohen said. This keeps the computer current and helps it refine its models further.
Business rules
Perhaps more importantly, Tractable pairs the lessons that the AI’s learned from the photo and estimate data set with a set of business rules “which is not AI,” according to Cohen.
This can control for factors which wouldn’t be visible to the computer. Cohen offered this example: The AI scores a part as a low-severity, repairable 4. But its business rules flag that the part is made out of plastic or aluminum — so it’s replace-only.
Cohen said these business rules are “customer-specific.” He couldn’t elaborate much, calling it Tractable’s “secret sauce,” but they typically are generated by a large repairer and a large insurer in collaboration and can even be market-specific.
“That’s the easy part,” he said. The image recognition capability was the difficult part.
We asked why the insurer or repairer’s views mattered at all. Why not go straight to the OEM, which logically and in the eyes of the court knows better than either industry how its cars should be repaired?
Cohen said that while OEMs have the repair procedures, they don’t have rules in place for evaluating visual damage to a vehicle like those it was developing with insurers and repairers. He also said that as a startup, Tractable had to choose where to allocate its resources, settling on body shops and insurers.
“Tomorrow, we might be working with OEMs,” he said. He also pointed out that Tractable started in Europe, where OEMs have a different relationship with shops than in the U.S. (Unlike in the “right to repair” U.S., shops in the United Kingdom must rely on Thatcham to provide repair procedures, for example.)
The guys working with Tractable on creating the rules aren’t going to be the kind of low-level, fresh-out-of-training adjusters who misunderstand company policy or OEM procedures, we suggested, and Cohen agreed. (Or, conversely, the kind of repairers hacking their way through cars to make more hours and profit.) The company would be working with higher-level employees with presumably a better understanding of modern repair complexity and company policy. That could shape AI outputs more acceptable to a shop and customer and cut down on the friction seen today.
Tractable also ensures quality control by measuring the agreement rates between experts on the extent of the damage shown in the photograph, according to Cohen. The AI itself produces an internal confidence score — if it’s too uncertain, the images can be kicked over to a human.
Quality control
CEO Alexandre Dalyac said during TU-Automotive’s Connected Car Insurance USA last month that insurance experts typically see an agreement rate of 75-80 percent, but Tractable’s AI is usually 2-3 percentage points higher. He said the AI is only uncertain in about 8 percent of cases — and that number is dropping, he said.
Granted, variations of many of the issues we raised could apply to a human photo appraiser as well. In that regard, Tractable’s AI could be considered as good as one — and possibly even better on neutrality grounds.
“We are currently at human performance,” Cohen said. But unlike a desk reviewer who can be tempted to lowball a claim to meet some sort of financial target, the computer is unbiased, Cohen said.
Cohen said that’s “why I really like this technology,” describing it as “the objectivity and the consistency of AI versus the subjectivity of the desk.”
In that vein, we were impressed to see certain parts flagged as “replace” by the Tractable AI in a demo (see the videos), in contrast to the desire of some shops and insurers to repair components they shouldn’t.
Get ready
Tractable’s technology is very likely going to be the future of photo estimating, so shops need to prepare themselves and their customers for how to handle it.
Educate customers so they’re not just taking the initial estimate as Gospel. While Tractable is working on predictive analytics to take into account hidden damage — electronics concealed underneath exterior parts, or parts which seem OK but felt the crash pulse — Cohen agreed that supplements were likely going to be necessary for a while.
Therefore, write correct, thorough supplemental estimates adhering to OEM procedures to better refine the AI and business rules and because you should have been doing that anyway. The estimate generated by an adjuster, desk reviewer or Tractable’s AI isn’t your repair plan, after all. It’s a way for an insurer to triage cars and estimate how much money to set aside for the claim.
Finally, be prepared for individual insurers using the system differently — and the headaches this could produce. As discussed above, Tractable’s business rules are tailored to a particular customer — i.e., the insurer. And at Connected Cars USA, Dalyac was asked about about a scenario where a policyholder doesn’t have the option to argue with the AI. He speculated it would be up to an individual insurer to decide when such a “‘computer says no'” declaration would annoy a customer and when it wouldn’t, but overall, the speed of an AI claims experience would be a hit with consumers.
Correction: An earlier version of this report incorrectly identified Chief Commercial Officer Adrien Cohen’s title. The article has since been updated with the correct information.
More information:
Mitchell, Oct. 10-11, 2016
“Tractable presents at ITC 2015 (Insurance Technology Congress)”
Insurance Technology Congress London via YouTube, Jan. 7, 2016
Images:
Tractable’s AI in a demo classifies parts as repair, replace, or leave alone based on a severity score of the scratches and more serious damage. (John Huetter/Repairer Driven News)
Tractable Chief Commercial Officer Adrien Cohen discusses the AI photo-estimating system during a July 27, 2017, interview at NACE. (John Huetter/Repairer Driven News)